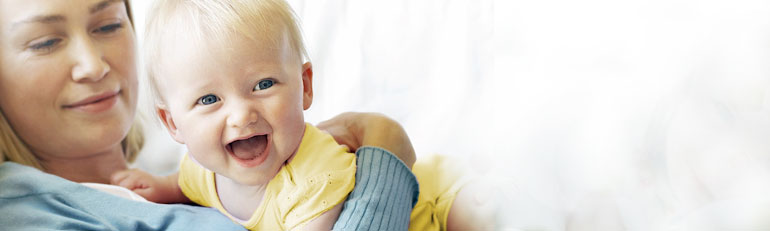
Material and Methods
Methods scheme was designed with base on three major tasks, above extensively described: Data acquisition, Algorithms Calculation, Validity Statistical Assessment.
Strategy was mainly defined attending a parallel study, with the same thematic and analytical bases, conducted in the United Kingdom11 and other prognostic scores validation studies conducted in Portugal12 [e.g. APPACHE (Acute Physiology, Age, Chronic Health Evaluation), SABS (Clinical Risk Index for Babies)].
1) Data acquisition
Data was acquired from a database previously created in the context of the precursor project Development and assessment of optimal risk scores for outcomes in paediatric intensive care (DAIP-CIP), extensively described before.
History of creation of database indicates prospective data collection in 3 volunteers Portuguese PICUs (Hospital Pediátrico de Coimbra - Coimbra, Hospital D. Estefânia - Lisbon, Hospital São João - Oporto), during a period of collection of 30 months. Collected data include data for all necessary variables used for the calculation of PRISM, PRISM III, PIM and PIM 2 (through routinely collection performed by health professionals and added specific pro-form, not routinely preconized). All admissions between 29 days and 16 years old were included in a total of 1809 admissions, without any more inclusion/exclusion criteria known. Data analysis of inter-observer, conducted in the second quarter of data collection, was performed in the way of data collection method’s quality assessment.
2) Algorithms calculation
Predicted probability of PICU mortality was calculated using the published algorithms for PIM, PIM2, PRISM and PRISM III.
3) Statistical Analysis
PASW Statistics18.0 was used for the statistical analysis. Performance of mortality risk scores were evaluated by assessing algorithms discrimination and calibration, and by comparison of observed and expected number of deaths through Standardized Mortality Ratio (SMR) analysis. Measuring the area under the Receiver Operating Characteristic (ROC) curve assessed discrimination. Hosmer and Lemeshow goodness-of-fit chi-square test assessed calibration. In descriptive Statistical analysis data are presented as mean±standard deviation. In inferential statistics 0.05 was set as significance level.13
Standardized Mortality Ratio (SMR)
An indirect mean of adjusting a rate, Standardized Mortality Ratio (SMR) is, commonly, defined as a ratio of observed deaths to expected deaths according to a specific health outcome. It is often used for comparing the observed mortality with the expected mortality would occur if the standard rates were applied.
The SMR may well be quoted with an indication of the uncertainty associated with its estimation, such as a confidence interval (CI) or p-value, which allows it to be interpreted in terms of statistical significance.
In clinical context, SMR is frequently used serving the comparative audit purpose of prediction systems, such as prognostic scores, in the way of evaluation of the services quality in clinical institutions. A SMR >1 usually reflects poor care.12
Discrimination
Discrimination is often defined as the ability of distinction between survivor and non-survivor. Predictions from each model are assessed using the c index (area under the receiver operating characteristic curve) for discrimination, which indicates, in fact, the probability of concordance between outcomes and predictions. In this study, it represents the probability that a randomly chosen patient who died will have a higher predicted probability of mortality than a randomly chosen patient who survived.
Published c-index criteria suggest that an area under the curve of 0.70-0.79 represents acceptable discrimination, being good discrimination represented by an area higher than 0.80. Excellent discriminatory power is represented by an area under the curve higher than 0.913.
Calibration
Calibration measures the correlation between the predicted outcomes and actual outcome over the entire range of risk prediction, this is, how well the predicted probabilities of mortality that were generated by the risk-adjustment models compared with the observed mortality will be assessed using the Hosmer-Lemeshow test. For each risk-adjustment model, for the Hosmer-Lemeshow goodness-of-fit chi square test, patients were categorized into 10 groups (eventually less) according to quintiles of their associated predicted probability of mortality, and the observed and expected outcomes were compared using a chi-square statistic.
Interpretation of Hosmer-Lemeshow goodness of fit test shows that if the difference between the observed and expected mortality is not significant, then they are comparable and the model has a significantly good calibration. Perfect calibration would be indicated by a model with a constant term of 0 and a slope term of 1. Significant differences from these values give a quantifiable indication of where the calibration of the models has failed. If the model predicts well, the events will be concentrated in the highest risk groups.13
In present study, Hosmer-Lemeshow goodness of fit test was applied in the way of score’s calibration evaluation in five categories of expected mortality probability (namely <1%; [1,5[%;[ 5,15[%; [15,30[%; >30%).15
4) Optimization of PIM2 current model
Attending to the poor calibration revealed by PIM2 in Portuguese data, a first-level customization, in the way of its optimization for a better fitting in Portuguese reality, was performed. Logistic regression on the original score, with base on Portuguese patients data, was made and the corresponding probability of PICU death was calculated for the customized score (C-PIM2). Calibration and discrimination were assessed, on the development sample, in the customized model, as previously described for the original models.